来源: Gpt进阶(二):训练部署自己的ChatGPT模型(羊驼 Alpaca-LoRA) – 知乎
参考:
1 Alpaca-LoRA(羊驼模型):
http://github.com/tloen/alpaca-lora
2 Chinese-alpaca-lora(开源的中文数据集)
http://github.com/LC1332/Chinese-alpaca-lora/blob/main/data/trans_chinese_alpaca_data.json
3 lora(git和论文参考):https://github.com/microsoft/LoRA
本文重要介绍使用羊驼模型,在消费级显卡中,几小时内就可以完成Alpaca的微调工作
我们目前使用的都是openai的模型接口,出于数据安全的考虑,我们有时候也需要部署一个自己的AI模型,但GPT并没有开源相关模型的代码,想训练自己的模型,只能找到一些开源算法模型微调后,训练属于自己的gpt模型
LLM的开源社区贡献了很多可以给我们自己训练的模型。比如Meta开源了对标GPT3模型的LLaMA模型,斯坦福在其基础上进行微调,得到了Alpaca模型,也就是目前比较火的羊驼
lora:大型语言模型的低秩适配器;简单来说就是微调模型的另一种方式,来调试模型在具体场景下的准确度;假设模型适应过程中的权重变化也具有较低的“内在秩”,从而提出了低秩适应(low – rank adaptation, LoRA)方法。论文地址(github):https://github.com/microsoft/LoRA
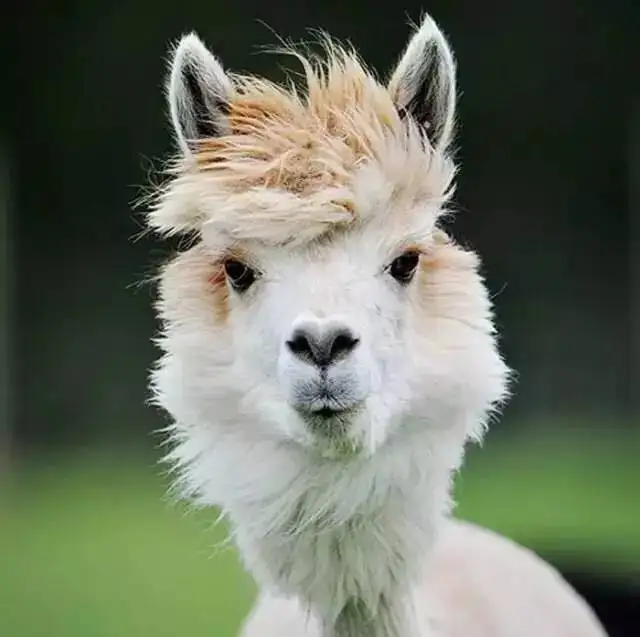
环境准备:
1 python环境
#网址:https://conda.io/en/latest/miniconda.html
wget https://repo.anaconda.com/miniconda/Miniconda3-py310_23.1.0-1-Linux-x86_64.sh #下载脚本
sh Miniconda3-py39_4.12.0-Linux-x86_64.sh # 执行
~/miniconda3/bin/conda init #初始化Shell,以便直接运行conda
conda create --name alpaca python=3.9 #关启shell,创建虚拟环境
conda activate alpaca #激活
2 下载羊驼代码
git clone https://github.com/tloen/alpaca-lora.git #下载源代码
cd alpaca-lora
pip install -r requirements.txt #安装依赖
#测试pytorch
import torch
torch.cuda.is_available()
3 准备数据集
构造指令数据集结构,类似于instruct的方法

可参考使用开源的中文数据集:Chinese-alpaca-lora,链接开头已经给出,下载后放到项目根目录下
4 下载LLaMA基础模型
http://huggingface.co/decapoda-research/llama-7b-hf/tree/main
下载完成后放到根目录下/llama-7b-hf
训练模型
python finetune.py \
--base_model 'llama-7b-hf' \
--data_path './trans_chinese_alpaca_data.json' \
--output_dir './lora-alpaca-zh'
其他具体参数可以git链接
模型训练后, lora-alpaca-zh 下就有模型生成了
模型推理
Inference (generate.py)
python generate.py \
--load_8bit \
--base_model 'decapoda-research/llama-7b-hf' \
--lora_weights 'tloen/alpaca-lora-7b'
云端部署
使用kaggle部署模型,访问web交互
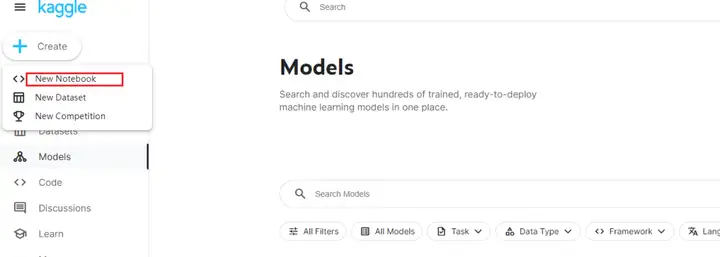
当然具体也可以部署到自己的服务器上。。。
最后,加入我们
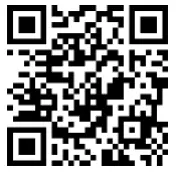